How Intelligent Tutoring Systems could change the way we learn
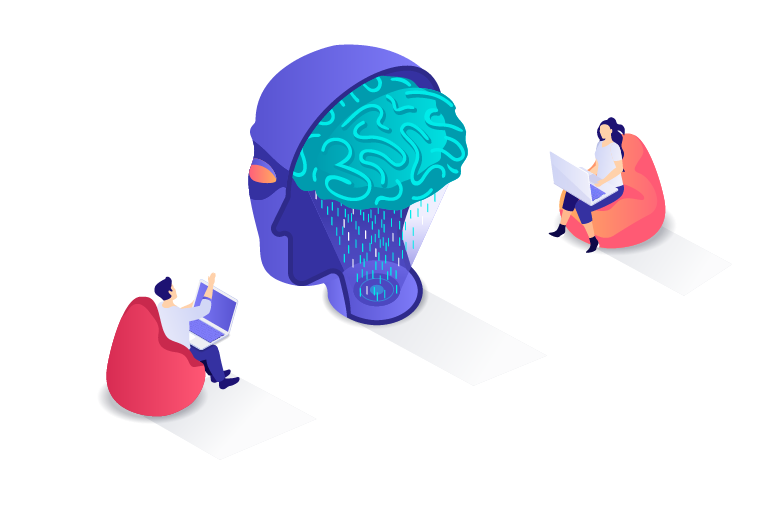
A Bit of History
Based primarily on Applications of AI in Education (1996), we will be exploring how computers have influenced education prior to 1996 in order to gain context into the history of Intelligent Tutoring Systems (ITSs). The next post in this line will be about the issues teachers found with modern ITSs when interfacing with them and the classroom. In addition to the historical perspective from Joseph Beck, Mia Stern and Erik Haugsjaa, I will be adding some perspective on the new technologies I have seen come in and out of fashion over the last 18 years, both intelligent and non-intelligent..
The history of computers in education began with simple step-by-step education in the forms of computer-aided instruction and computer-based training. Nowadays, computer-based learning is a cornerstone of most approaches to education. While a textbook still reigns supreme in a classroom, they are augmented with online learning across a range of platforms. However, many of the issues that ITSs were poised to solve from these early systems, have prevailed into the newer technologies used in modern day education.
With the rise of online learning tools, and the likes of M.O.O.Cs (massive open online learning courses), I would have expected to see many of the principles of ITSs creep into the design of modern systems and look less like the systems of the past and more like the ITSs envisioned at the fields inception. Some systems do incorporate some level of personalisation for a learner, such as Duolingo — as a well known example. However, this is only for one domain and only optimises difficulty for the learner. I will discuss these points later once we have a lens of what an ITS is.
Representation of a System
Below is a flow diagram of a conceptual ITS. If only things were this simple, everything would be so easy to learn.

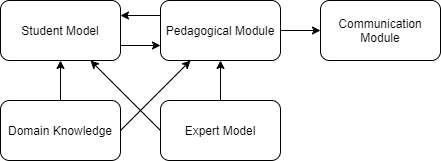
Fig 1. Beck, Stern and Haugsjaa’s interpretation of ITS component interactions
In brief, the Student Model is a component which collects data on the student, their learning style, acquisition and retention of knowledge over different concepts and topics and provides this information to the Domain Knowledge component and Pedagogical Module. The difficulty with this component is that there is a plethora of information which could be stored about the student depending on the capability of a particular ITS. There is a balance required to keep what is necessary and what could be useful for future development of the system and discarding excess information.
The Pedagogical Module is used to decide how a given learner should be taught a particular domain. This is dynamic meaning that if a learner is slower at learning a particular topic than the ITS predicted, the content will be scaled back to adapt to this. The Pedagogical Module is responsible for making decisions regarding the topic, feedback and problems a given learner sees. It uses the information provided to it by the Student model to build and execute a meta-strategy for teaching a particular domain. Feedback is also important, being able to determine when a learner is getting frustrated with a problem and giving them the right amount of feedback to progress without giving them too much information so they do not learn from a particular mistake.
The Domain Knowledge is combined with the Expert Module in most ITSs. However, in Applications of AI in Education, they chose to separate them to discuss their differences and particular functions. Both of these components need to contain all of the information a learner is being taught for a given topic.
The Domain Knowledge module is a reference to the information being taught to the learner. It requires knowledge engineering to communicate what information the learner needs to learn for a particular domain.
This information is then consumed by the Expert Module, which will normally take the form of a runnable expert model, able to compare the optimal solution with a student’s given solution and locate mistakes or points of friction for a learner. It also allows the Communication Module to interrupt at the time of a mistake if the Pedagogical Module deems that this is necessary for a given learner.
The last component of our conceptual ITS is the Communications Module. This is interfacing the ITS with the learned and deciding how to present information for the student to learn, being informed by the Pedagogical module how a student best learns and applying the presentation of the information for the student. This includes, but is not limited to the screen layout, interactions with the student, and whether to allow a student to request a hint or not.
What has happened in my lifetime and where I see ITSs having an impact
In my lifetime, I have used a range of learning tools, from Mathletics back in 2003 (It’s still active today) to Duolingo and Language Perfect for learning languages, Khan Academy, Brilliant, and so many more. In addition, I have seen the rise of M.O.O.Cs with the likes of EDx, Udemy, Coursera and Udacity come into play with many Universities throwing their hats in the ring. Many of these tools are great as an augmentation for learning and help learners with a range of styles to learn the content provided, but the one thing they all have in common is that their analytics for the learner and individualisation, in my experience, is minimal. Additionally, many of these courses will require a previous education and won’t necessarily teach the fundamentals of a subject, thereby being inaccessible to many around the world.
Many learning platforms have a suite of topics to learn from with Education Perfect ranging from mathematics to physical education and Duolingo having a suite of 19 distinct languages, Brilliant provides a huge range of STEM topics for a wide age range, and the list, once again, goes on. While this is undoubtedly a huge movement forward in educational technology, there is no system which does it all and can provide comprehensive feedback to teachers and parents on how students learn, what they are struggling with, what engages students, the trajectory of students learning, and a student’s engagement across a wide range of subjects.
The limit to this is also that students who are unable to attend school for whatever reason still fall behind on their content because they still require a teacher to teach the content. While I don’t believe ITSs should replace teachers due to the communication skills and interaction that a classroom provides, there should still be all the tools in place for students who are unable to attend school to get a comparable level and quality of education to those who can. This is where I foresee ITSs and educationally focussed technology having the biggest impact and positive effect on the current education practices.
Summarising
There are a range of education-focussed technologies available to augment the way learners learn today. However, many of these are still inaccessible by many potential learners around the world. ITSs were envisioned to be able to model a student’s learning and teach them at their own pace, in their own style. This would allow learners to learn the fundamentals and be informed around what is required to learn a new topic or skill and, where necessary, provide this to the learner before getting into a new topic. This allows education to be more accessible by everyone.
While it is great to see educational content become more and more open over my lifetime, there are still barriers which prevent it being accessible to everyone and there is potential for this to be solved by a new technology such as ITSs. Especially today, when countries around the world move in and out of lockdown, there is opportunity for complimentary systems to become established alongside traditional teaching, for those who find it difficult to learn over video conferencing applications.
About the author: Thomas Bingham is a Machine Learning Engineer on the Customer Success team at Arcanum. He found his way into the AI industry through studying Computer Engineering at the University of Canterbury with a twist of AI and ML papers which sparked his interest in this area.